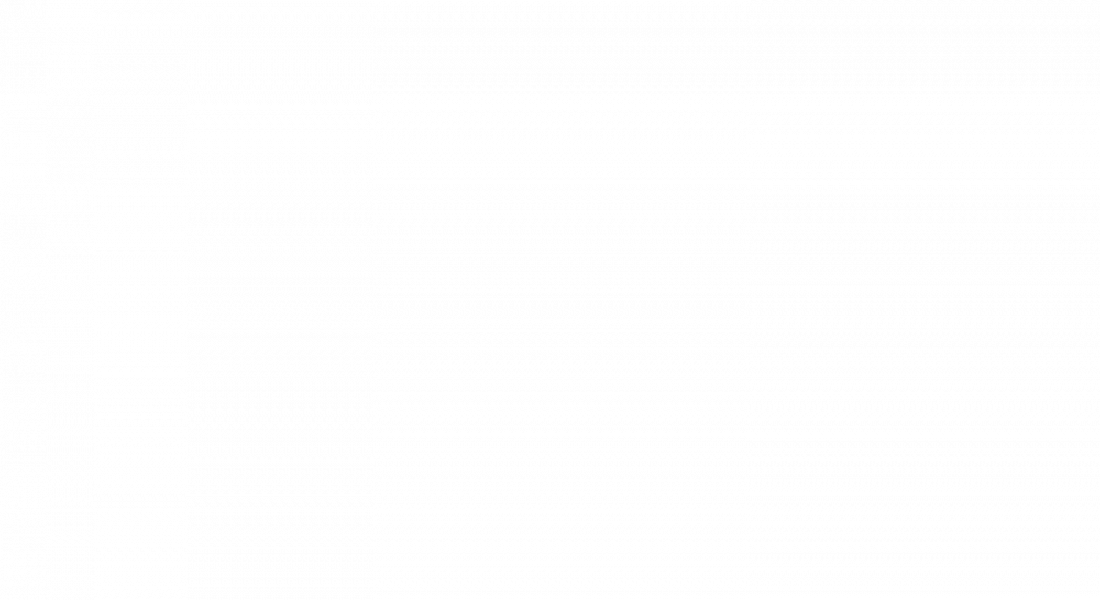
Can Machine Learning Predict Student Outcomes?
Ever wondered if machine learning can foresee how students will perform academically? Let’s dive into how this tech wizardry works!
- Machine learning helps schools spot students who might need extra help early on.
- It customises learning based on each student’s strengths and weaknesses.
- It uses concrete data to boost overall academic performance.
- But wait, it’s not all roses; there are some concerns too.
Predicting student performance is crucial for educational institutions aiming to enhance learning outcomes and provide timely support. Anticipating academic challenges and identifying at-risk students early allows schools to implement targeted interventions to improve success rates.
Machine learning leverages vast amounts of educational data to uncover patterns and insights traditional analysis might miss. This technology can improve student outcomes by making effective data-driven decisions.
Machine learning uses various data types to predict student performance, including grades, attendance records, participation metrics, socioeconomic background, and behavioural information. Cleaning and preparing data to eliminate errors is the first step. Developers then identify study habits and engagement levels to build predictive models using algorithms like decision trees and neural networks.
The early intervention enabled by machine learning allows schools to allocate resources effectively, addressing issues before they become critical. Recognizing potential academic struggles lets educators intervene with personalized strategies and assistance early.
Machine learning tailors educational experiences to individual needs, boosting student motivation and engagement. Analysing each student’s strengths, weaknesses, and learning preferences means these systems can customize lesson plans to their unique requirements. This personalized approach makes learning more relevant and accessible.
Machine learning provides data-driven strategies for boosting student achievement, enabling educators to implement effective interventions based on solid evidence. For example, students who complete their assigned tasks demonstrate a 3.4% increase in persistence rates. This showcases the impact of targeted support.
Ensuring high data quality is essential as the quality of predictions depends on it. Ongoing data quality management is necessary to maintain accurate and reliable results. Inaccurate information can negatively impact decision-making and the effectiveness of educational interventions.
Using machine learning to predict student performance carries the risk of sensitive data being mishandled or exposed. Educational institutions must implement advanced security protocols to protect student information against evolving cyber threats.
Bias and fairness are significant concerns in education when using machine learning. In 2023, 63% of U.S. adults expressed worries about AI bias and discrimination. Minimizing bias is essential to promote fairness and equity in educational predictions.
Balancing ethics and innovation in education requires human oversight alongside automated systems. Educators and policymakers must collaborate to establish guidelines that promote the ethical use of machine learning, ensuring it benefits students without compromising their welfare.
Balancing the benefits and challenges of machine learning in education ensures it is used ethically and effectively to support student success.
Source: Datasciencecentral